Gt 1030 gearforce software new free download 32 bit - apologise, but
Smart Grid and NFPA Electrical Safety Codes and Standards
Description:
Date uploaded
Copyright
Available Formats
Share this document
Share or Embed Document
Did you find this document useful?
Description:
Copyright:
Available Formats
Description:
Copyright:
Available Formats
Qun Niu · Zhile Yang · Patrick Luk (Eds.)
Communications in Computer and Information Science 763
Advanced Computational Methods
in Energy, Power, Electric Vehicles,
and Their Integration
International Conference on Life System Modeling
and Simulation, LSMS 2017
and International Conference on Intelligent Computing
for Sustainable Energy and Environment, ICSEE 2017
Nanjing, China, September 22–24, 2017, Proceedings, Part III
123
Communications
in Computer and Information Science 763
Commenced Publication in 2007
Founding and Former Series Editors:
Alfredo Cuzzocrea, Orhun Kara, Dominik Ślęzak, and Xiaokang Yang
Editorial Board
Simone Diniz Junqueira Barbosa
Pontifical Catholic University of Rio de Janeiro (PUC-Rio),
Rio de Janeiro, Brazil
Phoebe Chen
La Trobe University, Melbourne, Australia
Xiaoyong Du
Renmin University of China, Beijing, China
Joaquim Filipe
Polytechnic Institute of Setúbal, Setúbal, Portugal
Igor Kotenko
St. Petersburg Institute for Informatics and Automation of the Russian
Academy of Sciences, St. Petersburg, Russia
Ting Liu
Harbin Institute of Technology (HIT), Harbin, China
Krishna M. Sivalingam
Indian Institute of Technology Madras, Chennai, India
Takashi Washio
Osaka University, Osaka, Japan
More information about this series at http://www.springer.com/series/7899
Kang Li Yusheng Xue Shumei Cui
• •
Qun Niu Zhile Yang Patrick Luk (Eds.)
• •
Advanced Computational Methods
in Energy, Power, Electric Vehicles,
and Their Integration
International Conference on Life System Modeling
and Simulation, LSMS 2017
and International Conference on Intelligent Computing
for Sustainable Energy and Environment, ICSEE 2017
Nanjing, China, September 22–24, 2017
Proceedings, Part III
123
Editors
Kang Li Qun Niu
Queen’s University Belfast Shanghai University
Belfast Shanghai
UK China
Yusheng Xue Zhile Yang
Nanjing Automation Research Institute Queen’s University Belfast
Nanjing Belfast
China UK
Shumei Cui Patrick Luk
Harbin Institute of Technology Cranfield University
Harbin Bedford
China UK
ISSN 1865-0929 ISSN 1865-0937 (electronic)
Communications in Computer and Information Science
ISBN 978-981-10-6363-3 ISBN 978-981-10-6364-0 (eBook)
DOI 10.1007/978-981-10-6364-0
Library of Congress Control Number: 2017952379
© Springer Nature Singapore Pte Ltd. 2017
This work is subject to copyright. All rights are reserved by the Publisher, whether the whole or part of the
material is concerned, specifically the rights of translation, reprinting, reuse of illustrations, recitation,
broadcasting, reproduction on microfilms or in any other physical way, and transmission or information
storage and retrieval, electronic adaptation, computer software, or by similar or dissimilar methodology now
known or hereafter developed.
The use of general descriptive names, registered names, trademarks, service marks, etc. in this publication
does not imply, even in the absence of a specific statement, that such names are exempt from the relevant
protective laws and regulations and therefore free for general use.
The publisher, the authors and the editors are safe to assume that the advice and information in this book are
believed to be true and accurate at the date of publication. Neither the publisher nor the authors or the editors
give a warranty, express or implied, with respect to the material contained herein or for any errors or
omissions that may have been made. The publisher remains neutral with regard to jurisdictional claims in
published maps and institutional affiliations.
Printed on acid-free paper
This Springer imprint is published by Springer Nature
The registered company is Springer Nature Singapore Pte Ltd.
The registered company address is: 152 Beach Road, #21-01/04 Gateway East, Singapore 189721, Singapore
Preface
This book constitutes the proceedings of the 2017 International Conference on Life
System Modeling and Simulation (LSMS 2017) and the 2017 International Conference
on Intelligent Computing for Sustainable Energy and Environment (ICSEE 2017),
which were held during September 22–24, in Nanjing, China. These two international
conference series aim to bring together international researchers and practitioners in the
fields of advanced methods for life system modeling and simulation as well as
advanced intelligent computing theory and methodologies and engineering applications
for sustainable energy and environment. The two conferences held this year were built
on the success of previous LSMS and ICSEE conferences held in Shanghai and Wuxi,
respectively. The success of the LSMS and ICSEE conference series were also based
on several large-scale RCUK/NSFC funded UK–China collaborative projects on sus-
tainable energy and environment, as well as a recent government funded project on the
establishment of the UK-China University Consortium in Engineering Education and
Research, with an initial focus on sustainable energy and intelligent manufacturing.
At LSMS 2017 and ICSEE 2017, technical exchanges within the research com-
munity took the form of keynote speeches, panel discussions, as well as oral and poster
presentations. In particular, two workshops, namely, the Workshop on Smart Grid and
Electric Vehicles and the Workshop on Communication and Control for Distributed
Networked Systems, were held in parallel with LSMS 2017 and ICSEE 2017, focusing
on the two recent hot topics on green and sustainable energy systems and electric
vehicles and distributed networked systems for the Internet of Things.
The LSMS 2017 and ICSEE 2017 conferences received over 625 submissions from
14 countries and regions. All papers went through a rigorous peer review procedure and
each paper received at least three review reports. Based on the review reports, the
Program Committee finally selected 208 high-quality papers for presentation at LSMS
2017 and ICSEE 2017. These papers cover 22 topics, and are included in three volumes
of CCIS proceedings published by Springer. This volume of CCIS includes 79 papers
covering 7 relevant topics.
Located at the heartland of the wealthy lower Yangtze River region in China and
being the capital of several dynasties, kingdoms, and republican governments dating
back to the 3rd century, Nanjing has long been a major center of culture, education,
research, politics, economy, transport networks, and tourism. In addition to academic
exchanges, participants were treated to a series of social events, including receptions
and networking sessions, which served to build new connections, foster friendships,
and forge collaborations. The organizers of LSMS 2017 and ICSEE 2017 would like to
acknowledge the enormous contribution of the Advisory Committee, who provided
guidance and advice, the Program Committee and the numerous referees for their
efforts in reviewing and soliciting the papers, and the Publication Committee for
their editorial work. We would also like to thank the editorial team from Springer for
their support and guidance. Particular thanks are of course due to all the authors, as
VI Preface
without their high-quality submissions and presentations the conferences would not
have been successful.
Finally, we would like to express our gratitude to our sponsors and organizers, listed
on the following pages.
September 2017 Bo Hu Li
Sarah Spurgeon
Mitsuo Umezu
Minrui Fei
Kang Li
Dong Yue
Qinglong Han
Shiwei Ma
Luonan Chen
Sean McLoone
Organization
Sponsors
China Simulation Federation (CSF), China
Chinese Association for Artificial Intelligence (CAAI), China
IEEE Systems, Man & Cybernetics Society Technical Committee on Systems Biology,
USA
IEEE CC Ireland Chapter, Ireland
Technical Support Organization
National Natural Science Foundation of China (NSFC), China
Organizers
Shanghai University, China
Queen’s University Belfast, UK
Nanjing University of Posts and Telecommunications, China
Southeast University, China
Life System Modeling and Simulation Technical Committee of CSF, China
Embedded Instrument and System Technical Committee of China Instrument
and Control Society, China
Intelligent Control and Intelligent Management Technical Committee of CAAI, China
Co-sponsors
Shanghai Association for System Simulation, China
Shanghai Association of Automation, China
Shanghai Instrument and Control Society, China
Jiangsu Association of Automation, China
Co-organizers
Swinburne University of Technology, Australia
Queensland University of Technology, Australia
Tsinghua University, China
Harbin Institute of Technology, China
China State Grid Electric Power Research Institute, China
Chongqing University, China
University of Essex, UK
Cranfield University, UK
Peking University, China
VIII Organization
Nantong University, China
Shanghai Dianji University, China
Jiangsu Engineering Laboratory of Big Data Analysis and Control for Active
Distribution Network, China
Shanghai Key Laboratory of Power Station Automation Technology, China
Honorary Chairs
Li, Bo Hu, China
Spurgeon, Sarah, UK
Umezu, Mitsuo, Japan
Advisory Committee Members
Bai, Erwei, USA Nikolopoulos, Tan, KC, Singapore
Ge, Shuzhi, Singapore Dimitrios S., UK Tassou, Savvas, UK
He, Haibo, USA Pardalos, Panos M., USA Thompson, Stephen, UK
Hu, Huosheng, UK Pedrycz, Witold, Canada Wang, Jun, HK
Huang, Biao, Canada Polycarpou, Marios M., Wang, Zidong, UK
Hussain, Amir, UK Cyprus Wu, Qinghua, China
Liu, Derong, USA Qin, Joe, HK Xue, Yusheng, China
Mi, Chris, USA Scott, Stan, UK Zhang, Lin, China
General Chairs
Fei, Minrui, China
Li, Kang, UK
Yue, Dong, China
International Program Committee
Chairs
Chen, Luonan, Japan
Han, Qinglong, Australia
Ma, Shiwei, China
McLoone, Sean, UK
Local Chairs
Chiu, Min-Sen, Singapore Fridman, Emilia, Israel Hunger, Axel, Germany
Cui, Shumei, China Gao, Furong, HK Lam, Hak-Keung, UK
Deng, Mingcong, Japan Gu, Xingsheng, China Liu, Wanquan, Australia
Ding, Yongsheng, China Guerrero, Josep M., Luk, Patrick, UK
Ding, Zhengtao, UK Demark Maione, Guido, Italy
Fang, Qing, Japan Gupta, Madan M., Canada Park, Jessie, Korea
Organization IX
Peng, Chen, China Yang, Taicheng, UK Zhang, Jianhua, China
Su, Zhou, China Yu, Wen, Mexico Zhang, Wenjun, Canada
Tian, Yuchu, Australia Zeng, Xiaojun, UK Zhao, Dongbin, China
Xu, Peter, New Zealand Zhang, Huaguang, China
Members
Andreasson, Stefan, UK Han, Bo, China Liu, Shujun, China
Adamatzky, Andy, UK Han, Xuezheng, China Liu, Tingzhang, China
Altrock, Philipp, USA Heiland, Jan, Germany Liu, Xianzhong, China
Asirvadam, Vijay S., Hong, Xia, UK Liu, Yang, China
Malaysia Hou, Weiyan, China Liu, Yunhuai, China
Baig, Hasan, UK Hu, Liangjian, China Liu, Zhen, China
Baker, Lucy, UK Hu, Qingxi, China Ljubo, Vlacic, Australia
Barry, John, UK Hu, Sideng, China Lu, Ning, Canada
Best, Robert, UK Huang, Sunan, Singapore Luan, Tom, Australia
Bu, Xiongzhu, China Huang, Wenjun, China Luo, Jianfei, China
Cao, Jun, UK Hwang, Tan Teng, Ma, Hongjun, China
Cao, Yi, UK Malaysia McAfee, Marion, Ireland
Chang, Xiaoming, China Jia, Dongyao, UK Menary, Gary, UK
Chen, Jing, China Jiang, Lin, UK Meng, Xianhai, UK
Chen, Ling, China Jiang, Ming, China Menhas, Muhammad
Chen, Qigong, China Jiang, Ping, China Ilyas, Pakistan
Chen, Rongbao, China Jiang, Yucheng, China Menzies, Gillian, UK
Chen, Wenhua, UK Kuo, Youngwook, UK Naeem, Wasif, UK
Cotton, Matthew, UK Laverty, David, UK Nie, Shengdong, China
Deng, Jing, UK Li, Chuanfeng, China Niu, Yuguang, China
Deng, Li, China Li, Chuanjiang, China Nyugen, Bao Kha, UK
Deng, Shuai, China Li, Dewei, China Ouyang, Mingsan, China
Deng, Song, China Li, Donghai, China Oyinlola, Muyiwa, UK
Deng, Weihua, China Li, Guozheng, China Pan, Hui, China
Ding, Yate, UK Li, Jingzhao, China Pan, Ying, China
Ding, Zhigang, China Li, Ning, China Phan, Anh, UK
Du, Dajun, China Li, Tao, China Qadrdan, Meysam, UK
Du, Xiangyang, China Li, Tongtao, China Qian, Hua, China
Ellis, Geraint, UK Li, Weixing, China Qu, Yanbin, China
Fang, Dongfeng, USA Li, Xin, China Raszewski, Slawomir, UK
Feng, Dongqing, China Li, Xinghua, China Ren, Wei, China
Feng, Zhiguo, China Li, Yunze, China Rivotti, Pedro, UK
Foley, Aoife, UK Li, Zhengping, China Rong, Qiguo, China
Fu, Jingqi, China Lin, Zhihao, China Shao, Chenxi, China
Gao, Shouwei, China Lino, Paolo, Italy Shi, Yuntao, China
Gu, Dongbin, UK Liu, Chao, France Smyth, Beatrice, UK
Gu, Juping, China Liu, Guoqiang, China Song, Shiji, China
Gu, Zhou, China Liu, Mandan, China Song, Yang, China
Guo, Lingzhong, UK Liu, Shirong, China Su, Hongye, China
X Organization
Sun, Guangming, China Wu, Lingyun, China Yue, Hong, UK
Sun, Xin, China Wu, Zhongcheng, China Zeng, Xiaojun, UK
Sun, Zhiqiang, China Xie, Hui, China Zhang, Dengfeng, China
Tang, Xiaoqing, UK Xu, Sheng, China Zhang, Hongguang, China
Teng, Fei, UK Xu, Wei, China Zhang, Jian, China
Teng, Huaqiang, China Xu, Xiandong, UK Zhang, Jingjing, UK
Trung, Dong, UK Yan, Huaicheng, China Zhang, Lidong, China
Tu, Xiaowei, China Yan, Jin, UK Zhang, Long, UK
Vlacic, Ljubo, UK Yang, Aolei, China Zhang, Qianfan, China
Wang, Gang, China Yang, Kan, USA Zhang, Xiaolei, UK
Wang, Jianzhong, China Yang, Shuanghua, UK Zhang, Yunong, China
Wang, Jihong, UK Yang, Wankou, China Zhao, Dongya, China
Wang, Ling, China Yang, Wenqiang, China Zhao, jun, China
Wang, Mingshun, China Yang, Zhile, UK Zhao, Wanqing, UK
Wang, Shuangxin, China Yang, Zhixin, Macau Zhao, Xiaodong, UK
Wang, Songyan, China Ye, Dan, China Zhao, Xingang, China
Wang, Yaonan, China You, Keyou, China Zheng, Xiaojun, UK
Wei, Kaixia, China Yu, Ansheng, China Zhou, Huiyu, UK
Wei, Lisheng, China Yu, Dingli, UK Zhou, Wenju, China
Wei, Mingshan, China Yu, Hongnian, UK Zhou, Yu, China
Wen, Guihua, China Yu, Xin, China Zhu, Yunpu, China
Wu, Jianguo, China Yuan, Jin, China Zong, Yi, Demark
Wu, Jianzhong, UK Yuan, Jingqi, China Zuo, Kaizhong, China
Organization Committee
Chairs
Li, Xin, China
Wu, Yunjie, China
Naeem, Wasif, UK
Zhang, Tengfei, China
Cao, Xianghui, China
Members
Chen, Ling, China
Deng, Li, China
Du, Dajun, China
Jia, Li, China
Song, Yang, China
Sun, Xin, China
Xu, Xiandong, China
Yang, Aolei, China
Yang, Banghua, China
Zheng, Min, China
Zhou, Peng, China
Organization XI
Special Session Chairs
Wang, Ling, China
Meng, Fanlin, UK
Publication Chairs
Zhou, Huiyu, UK
Niu, Qun, China
Publicity Chairs
Jia, Li, China
Yang, Erfu, UK
Registration Chairs
Song, Yang, China
Deng, Li, China
Secretary-General
Sun, Xin, China
Wu, Songsong, China
Yang, Zhile, UK
Contents
Computational Intelligence in Utilization of Clean
and Renewable Energy Resources
Research on Wind Speed Vertical Extrapolation Based on Extreme
Learning Machine . . . . . . . . . . . . . . . . . . . . . . . . . . . . . . . . . . . . . . . . . . 3
Hui Lv and Guochu Chen
Optimal Scheduling of Wind Turbine Generator Units Based
on the Amount of Damage of Impeller . . . . . . . . . . . . . . . . . . . . . . . . . . . 12
Kai Lin and Guochu Chen
A Short Term Wind Speed Forecasting Method Using Signal
Decomposition and Extreme Learning Machine. . . . . . . . . . . . . . . . . . . . . . 22
Sizhou Sun, Jingqi Fu, and Feng Zhu
A Novel Method for Short-Term Wind Speed Forecasting Based
on UPQPSO-LSSVM . . . . . . . . . . . . . . . . . . . . . . . . . . . . . . . . . . . . . . . 32
Wangxue Nie, Jingqi Fu, and Sizhou Sun
Structure Design and Parameter Computation of a Seawater
Desalination System with Vertical Axis Wind Turbine. . . . . . . . . . . . . . . . . 43
Yihuai Hu, Kai Li, and Hao Jin
Inertial Response Control Strategy of Wind Turbine Based
on Variable Universe Fuzzy Control . . . . . . . . . . . . . . . . . . . . . . . . . . . . . 52
Le Gao, Guoxing Yu, Lan Liu, and Huihui Song
System Frequency Control of Variable Speed Wind Turbines
with Variable Controller Parameters . . . . . . . . . . . . . . . . . . . . . . . . . . . . . 63
Guoyi Xu, Chen Zhu, Libin Yang, Chunlai Li, Jun Yang, and Tianshu Bi
Base-Load Cycling Capacity Adequacy Evaluation in Power Systems
with Wind Power . . . . . . . . . . . . . . . . . . . . . . . . . . . . . . . . . . . . . . . . . . 74
Jingjie Ma, Shaohua Zhang, and Liuhui Wang
MFAC-PID Control for Variable-Speed Constant Frequency Wind Turbine . . . 84
Qingye Meng, Shuangxin Wang, Jianhua Zhang, and Tingting Guo
A Multivariate Wind Power Fitting Model Based on Cluster
Wavelet Neural Network . . . . . . . . . . . . . . . . . . . . . . . . . . . . . . . . . . . . . 94
Ruiwen Zheng, Qing Fang, Zhiyuan Liu, Binghong Li,
and Xiao-Yu Zhang
XIV Contents
Control Strategy for Isolated Wind-Solar-Diesel Micro Grid System
Considering Constant Load . . . . . . . . . . . . . . . . . . . . . . . . . . . . . . . . . . . 103
Xuejian Yang, Dong Yue, and Tengfei Zhang
Equilibrium Analysis of Electricity Market with Wind Power Bidding
and Demand Response Bidding . . . . . . . . . . . . . . . . . . . . . . . . . . . . . . . . 111
Kai Zhang, Xian Wang, and Shaohua Zhang
Stability Analysis of Wind Turbines Combined with Rechargeable
Batteries Based on Markov Jump Linear Systems . . . . . . . . . . . . . . . . . . . . 126
Xiao-kun Dai, Yang Song, Mira Schüller, and Dieter Schramm
Modeling and Simulation Study of Photovoltaic DC Arc Faults . . . . . . . . . . 137
Zhihua Li, Zhiqun Ye, Chunhua Wu, and Wenxin Xu
Data Management of Water Flow Standard Device Based on LabVIEW . . . . 147
Shaoshao Qin, Bin Li, and Chao Cheng
Design and Research of Water Flow Standard Facilities Based
on Field Service . . . . . . . . . . . . . . . . . . . . . . . . . . . . . . . . . . . . . . . . . . . 157
Chao Cheng, Bin Li, and Shaoshao Qin
An Improved Multi-objective Bare-Bones PSO for Optimal Design
of Solar Dish Stirling Engine Systems . . . . . . . . . . . . . . . . . . . . . . . . . . . . 167
Qun Niu, Ziyuan Sun, and Dandan Hua
Fault Diagnosis Method of Ningxia Photovoltaic Inverter Based
on Wavelet Neural Network . . . . . . . . . . . . . . . . . . . . . . . . . . . . . . . . . . . 178
Guohua Yang, Pengzhen Wang, Bingxuan Li, Bo Lei, Hao Tang,
and Rui Li
Research on Expert Knowledge Base of Intelligent Diagnosis Based
on Tubing Leakage of High-Pressure Heater in Nuclear Power Plant . . . . . . . 185
Miao Zheng, Hong Qian, Siyun Lin, Bole Xiao, and Xiaoping Chu
Research on Intelligent Early-Warning System of Main Pipeline in Nuclear
Power Plants Based on Hierarchical and Multidimensional Fault
Identification Method. . . . . . . . . . . . . . . . . . . . . . . . . . . . . . . . . . . . . . . . 195
Hong Qian, Siyun Lin, Miao Zheng, and Qiang Zhang
The Early Warning System of Nuclear Power Station Oriented
to Human Reliability . . . . . . . . . . . . . . . . . . . . . . . . . . . . . . . . . . . . . . . . 206
Shuai Ren and Hong Qian
Research on Energy Interconnection Oriented Big Data Sharing
Platform Reference Architecture . . . . . . . . . . . . . . . . . . . . . . . . . . . . . . . . 217
Wei Rao, Jing Jiang, Ming Yang, Wei Peng, and Aihua Zhou
Contents XV
Intelligent Methods for Energy Saving and Pollution Reduction
Study on Lightweight Design and Connection of Dissimilar Metals
of Titanium Alloy TC4/T2 Copper/304 Stainless Steel . . . . . . . . . . . . . . . . . 229
Shun Guo, Qi Zhou, Peng Xu, Qiong Gao, Tianyuan Luo, Yong Peng,
Jian Kong, KeHong Wang, and Jun Zhu
Research on Warehouse Scheduling Optimization Problem
for Broiler Breeding . . . . . . . . . . . . . . . . . . . . . . . . . . . . . . . . . . . . . . . . 238
Wenqiang Yang and Yongfeng Li
A Discrete Fourier Transform Based Compensation Task Sharing
Method for Power Quality Improvement . . . . . . . . . . . . . . . . . . . . . . . . . . 247
Jianbo Chen, Dong Yue, Chunxia Dou, and Chongxin Huang
A Comprehensive Optimization of PDl Controller Design for Trade-off
of Energy and System Performance . . . . . . . . . . . . . . . . . . . . . . . . . . . . . . 257
Ke Zhang, Min Zheng, Kang Li, and Yijie Zhang
Hierarchical Time Series Feature Extraction for Power Consumption
Anomaly Detection . . . . . . . . . . . . . . . . . . . . . . . . . . . . . . . . . . . . . . . . . 267
Zhiyou Ouyang, Xiaokui Sun, and Dong Yue
Prospect Theory Based Electricity Allocation for GenCos Considering
Uncertainty of Emission Price. . . . . . . . . . . . . . . . . . . . . . . . . . . . . . . . . . 276
Yue Zhang and Shaohua Zhang
Neural-Network-Based Tracking Control of Offshore Steel Jacket Platforms . . . 287
Zhi-Hui Cai, Bao-Lin Zhang, and Xian-Hu Yu
Intelligent Methods in Developing Electric Vehicles, Engines and Equipment
Short-Term Optimal Scheduling with the Consideration of Electric
Vehicle Driving Rules . . . . . . . . . . . . . . . . . . . . . . . . . . . . . . . . . . . . . . . 299
Xiaolin Ge and Chenhao Pei
Dispatching Analysis of Ordered Charging Considering
the Randomness Factor of Electric Vehicles Charging . . . . . . . . . . . . . . . . . 309
Ling Mao and Enyu Jiang
A Contract Based Approach for Electric Vehicles Charging
in Heterogeneous Networks . . . . . . . . . . . . . . . . . . . . . . . . . . . . . . . . . . . 319
Huwei Chen, Zhou Su, Yilong Hui, Hui Hui, and Dongfeng Fang
Review of the Four Ports Electromechanical Converter Used
for Hybrid Electric Vehicle . . . . . . . . . . . . . . . . . . . . . . . . . . . . . . . . . . . 329
Qiwei Xu, Jing Sun, Meng Zhao, Xiaobiao Jiang, Yunqi Mao,
and Shumei Cui
XVI Contents
Research on Parameters Matching of Hybrid Electric Vehicle
with Compound-Structure Induction Machine . . . . . . . . . . . . . . . . . . . . . . . 339
Qiwei Xu, Xiaobiao Jiang, Meng Zhao, Xiaoxiao Luo, Weidong Chen,
Yunqi Mao, and Shumei Cui
Location Model Research of Charging Station for Electric Vehicle Based
on Users’ Benefit . . . . . . . . . . . . . . . . . . . . . . . . . . . . . . . . . . . . . . . . . . 351
Fei Xia, Zhicheng Wang, Daogang Peng, Zihao Li, Zhijiang Luo,
and Bo Yuan
Research on Double Fuzzy Control Strategy for Parallel Hybrid
Electric Bus . . . . . . . . . . . . . . . . . . . . . . . . . . . . . . . . . . . . . . . . . . . . . . 362
Qiwei Xu, Xiaoxiao Luo, Xiaobiao Jiang, and Meng Zhao
Optimal Battery Charging Strategy Based on Complex
System Optimization . . . . . . . . . . . . . . . . . . . . . . . . . . . . . . . . . . . . . . . . 371
Haiping Ma, Pengcheng You, Kailong Liu, Zhile Yang, and Minrui Fei
Experimental Research on Power Battery Fast Charging Performance . . . . . . 379
Jinlei Sun, Lei Li, Fei Yang, Qiang Li, and Chao Wu
A Novel RBF Neural Model for Single Flow Zinc Nickel Batteries. . . . . . . . 386
Xiang Li, Kang Li, Zhile Yang, and Chikong Wong
State-of-Charge Estimation of Lithium Batteries Using Compact RBF
Networks and AUKF. . . . . . . . . . . . . . . . . . . . . . . . . . . . . . . . . . . . . . . . 396
Li Zhang, Kang Li, Dajun Du, Minrui Fei, and Xiang Li
Intelligent Computing and Control in Power Systems
Design of Adaptive Predictive Controller for Superheated Steam
Temperature Control in Thermal Power Plant . . . . . . . . . . . . . . . . . . . . . . . 409
Hong Qian, Yu-qing Feng, and Zi-bin Zheng
Extended State Space Predictive Control of Gas Turbine System
in Combined Cycle Power Plant . . . . . . . . . . . . . . . . . . . . . . . . . . . . . . . . 420
Guolian Hou, Tian Wang, Huan Du, Jianhua Zhang, and Xiaobin Zheng
Decentralized H1 Load Frequency Control for Multi-area Power Systems
with Communication Uncertainties . . . . . . . . . . . . . . . . . . . . . . . . . . . . . . 429
Yanliang Cui, Guangtian Shi, Lanlan Xu, Xiaoan Zhang, and Xue Li
Cyber Security Against Denial of Service of Attacks on Load Frequency
Control of Multi-area Power Systems . . . . . . . . . . . . . . . . . . . . . . . . . . . . 439
Yubin Shen, Minrui Fei, Dajun Du, Wenjun Zhang, Srdjan Stanković,
and Aleksandar Rakić
Contents XVII
Detecting Replay Attacks in Power Systems: A Data-Driven Approach . . . . . 450
Mingliang Ma, Peng Zhou, Dajun Du, Chen Peng, Minrui Fei,
and Hanan Mubarak AlBuflasa
A Novel Dynamic State Estimation Algorithm in Power Systems
Under Denial of Service Attacks . . . . . . . . . . . . . . . . . . . . . . . . . . . . . . . . 458
Mengzhuo Yang, Xue Li, and Dajun Du
Small-Signal Refinement of Power System Static Load
Modelling Techniques . . . . . . . . . . . . . . . . . . . . . . . . . . . . . . . . . . . . . . . 467
Gareth McLorn and Seán McLoone
H∞ Prediction Triggering Control of Multi-area Power Systems Load
Frequency Control Under DoS Attacks . . . . . . . . . . . . . . . . . . . . . . . . . . . 477
Zihao Cheng, Dong Yue, Xinli Lan, Chongxin Huang, and Songlin Hu
New Framework Mining Algorithm Based Main Operation Parameters
Optimization in Power Plant . . . . . . . . . . . . . . . . . . . . . . . . . . . . . . . . . . . 488
Wencheng Huang, Li Jia, and Daogang Peng
A Consensus-Based Distributed Primal-Dual Perturbed Subgradient
Algorithm for DC OPF . . . . . . . . . . . . . . . . . . . . . . . . . . . . . . . . . . . . . . 497
Zhongyuan Yang, Bin Zou, and Junmeng Zhang
Model Predictive Control Based on the Dynamic PLS Approach
to Waste Heat Recovery System . . . . . . . . . . . . . . . . . . . . . . . . . . . . . . . . 509
Jianhua Zhang, Haopeng Hu, Jinzhu Pu, and Guolian Hou
Optimized Control of Ship DC Electric Propulsion System
with Energy Storage Unit. . . . . . . . . . . . . . . . . . . . . . . . . . . . . . . . . . . . . 519
Feng Ding, Shuofeng Wang, and Shaohua Zhang
The Application of the Particle Swarm Algorithm to Optimize PID
Controller in the Automatic Voltage Regulation System . . . . . . . . . . . . . . . . 529
Jing Wang, Naichao Song, Enyu Jiang, Da Xu, Weihua Deng,
and Ling Mao
Research on the Bio-electromagnetic Compatibility of Artificial Anal
Sphincter Based on Transcutaneous Energy Transfer . . . . . . . . . . . . . . . . . . 537
Peng Zan, Chundong Zhang, Suqin Zhang, Yankai Liu, and Yong Shao
The Role of Intelligent Computing in Load Forecasting for Distributed
Energy System . . . . . . . . . . . . . . . . . . . . . . . . . . . . . . . . . . . . . . . . . . . . 547
Pengwei Su, Yan Wang, Jun Zhao, Shuai Deng, Ligai Kang, Zelin Li,
and Yu Jin
XVIII Contents
Intelligent Control Methods of Demand Side Management in Integrated
Energy System: Literature Review and Case Study . . . . . . . . . . . . . . . . . . . 556
Yan Wang, Pengwei Su, Jun Zhao, Shuai Deng, Hao Li, and Yu Jin
Optimal Design and Operation of Integrated Energy System Based
on Supply-Demand Coupling Analysis. . . . . . . . . . . . . . . . . . . . . . . . . . . . 566
Qiong Wu and Hongbo Ren
Modeling, Simulation and Control in Smart Grid and Microgrid
Control Strategies for the Microgrid Control System
with Communication Delays. . . . . . . . . . . . . . . . . . . . . . . . . . . . . . . . . . . 579
Weihua Deng, Pengfei Chen, Kang Li, and Chuanfeng Li
Secondary Voltage Control of Microgrids with Distributed
Event-Triggered Mechanism . . . . . . . . . . . . . . . . . . . . . . . . . . . . . . . . . . . 587
Jing Shi, Dong Yue, and Shengxuan Weng
Frequent Deviation-Free Control for Micro-Grid Operation Modes
Switching Based on Virtual Synchronous Generator . . . . . . . . . . . . . . . . . . 597
Yan Xu, Tengfei Zhang, and Dong Yue
A Novel Data Injection Cyber-Attack Against Dynamic State Estimation
in Smart Grid . . . . . . . . . . . . . . . . . . . . . . . . . . . . . . . . . . . . . . . . . . . . . 607
Rui Chen, Dajun Du, and Minrui Fei
A Novel Combination of Forecasting Model Based on ACCQPSO-LSSVM
and Its Application . . . . . . . . . . . . . . . . . . . . . . . . . . . . . . . . . . . . . . . . . 616
Nan Xiong, Minrui Fei, Sizhou Sun, and Taicheng Yang
Research on Power Terminal Access Control Technology Supporting
Internet Interactive Service in Smart Grid. . . . . . . . . . . . . . . . . . . . . . . . . . 626
Song Deng, Liping Zhang, and Dong Yue
Research on Model and Method of Maturity Evaluation of Smart
Grid Industry . . . . . . . . . . . . . . . . . . . . . . . . . . . . . . . . . . . . . . . . . . . . . 633
Yue He, Junyong Wu, Yi Ge, Dezhi Li, and Huaguang Yan
An Improved Multi-objective Differential Evolution Algorithm
for Active Power Dispatch in Power System with Wind Farms . . . . . . . . . . . 643
Shu Xia, Yingcheng Xu, and Xiaolin Ge
Integration of the Demand Side Management with Active and Reactive
Power Economic Dispatch of Microgrids . . . . . . . . . . . . . . . . . . . . . . . . . . 653
Mohammed K. Al-Saadi, Patrick C.K. Luk, and John Economou
Contents XIX
Unit Commitment Dynamic Unified Active and Reactive Power Dispatch
of Microgrids with Integration of Electric Vehicles . . . . . . . . . . . . . . . . . . . 665
Mohammed K. Al-Saadi, Patrick C.K. Luk, and John Economou
Optimal Design and Planning of Electric Vehicles Within Microgrid . . . . . . . 677
Mohammed Alkhafaji, Patrick Luk, and John Economou
Security-Constrained Two-Stage Stochastic Unified Active and Reactive
Power Management System of the Microgrids . . . . . . . . . . . . . . . . . . . . . . 691
Mohammed K. Al-Saadi and Patrick C.K. Luk
Charging and Discharging Strategy of Electric Vehicles
Within a Hierarchical Energy Management Framework . . . . . . . . . . . . . . . . 704
Mohammed Alkhafaji, Patrick Luk, and John Economou
Optimization Methods
Optimization Allocation of Aerospace Ground Support Vehicles
for Multiple Types of Military Aircraft . . . . . . . . . . . . . . . . . . . . . . . . . . . 719
Fuqin Yang, Jinhua Li, and Mingzhu Zhu
Multi-level Maintenance Economic Optimization Model of Electric
Multiple Unit Component Based on Shock Damage Interaction. . . . . . . . . . . 729
Hong Wang, Yong He, Lv Xiong, and Zuhua Jiang
A Composite Controller for Piezoelectric Actuators with Model
Predictive Control and Hysteresis Compensation . . . . . . . . . . . . . . . . . . . . . 740
Ang Wang and Long Cheng
Computational Methods for Sustainable Environment
Numerical Investigation of the Environment Capacity of COD,
Inorganic Nitrogen and Phosphate in the Bohai Bay . . . . . . . . . . . . . . . . . . 753
Hao Liu and Zhi-kang Zhang
An Artificial Neural Network Model for Predicting Typhoon Intensity
and Its Application . . . . . . . . . . . . . . . . . . . . . . . . . . . . . . . . . . . . . . . . . 762
Ruyun Wang, Tian Wang, Xiaoyu Zhang, Qing Fang, Chumin Wu,
and Bin Zhang
Analysis of Power Spectrum Feature Based on Slurry Noise
in Electromagnetic Flowmeter. . . . . . . . . . . . . . . . . . . . . . . . . . . . . . . . . . 771
Jie Chen, Qiong Fei, Bin Li, and Xiaojie Zheng
A Two-Stage Agriculture Environmental Anomaly Detection Method . . . . . . 779
Lili Wang, Yue Yu, Li Deng, and Honglin Pang
XX Contents
Building a Virtual Reality System for Intelligent Agriculture
Greenhouse Based on Web3D . . . . . . . . . . . . . . . . . . . . . . . . . . . . . . . . . 790
Qun Huang, Li Deng, Minrui Fei, and Huosheng Hu
A Green Dispatch Model of Power System with Wind Energy
Considering Energy-Environmental Efficiency . . . . . . . . . . . . . . . . . . . . . . 800
Daojun Chen, Liqing Liang, Lei Zhang, Jian Zuo, Keren Zhang,
Chenkun Li, and Hu Guo
Author Index . . . . . . . . . . . . . . . . . . . . . . . . . . . . . . . . . . . . . . . . . . . . 813
Computational Intelligence in Utilization
of Clean and Renewable Energy
Resources
Research on Wind Speed Vertical
Extrapolation Based on Extreme
Learning Machine
Hui Lv and Guochu Chen(&)
Electric Engineering School,
Shanghai DianJi University, Shanghai 201306, China
770440679@qq.com, chengc@sdju.edu.cn
Abstract. In engineering, the method of wind speed vertical extrapolation is
based on the actual wind data of the wind tower, and the wind shear index is
used to calculate the wind speed at any height in the near ground. The wind
shear index is only considered in the neutral state of the atmosphere, without
considering the impact of atmospheric stability on the wind shear index, which
has some limitations. At the same time, the calculation of the wind shear index is
a rather complicated task when considering the atmospheric stability. In order to
solve these problems, this paper puts forward to use extreme learning machine
for fitting the relationship between wind speed at different heights. Extreme
learning machine has the advantages of fast learning speed, good generalization
ability and so on. In this paper, the results obtained by the extreme learning
machine and traditional methods are compared with the measured values. The
results show that the extreme learning machine has a better application prospect
in the vertical wind speed extrapolation.
Keywords: Extreme learning machine Wind speed Vertical extrapolation
Wind shear index
1 Introduction
The accurate calculation of the wind resource data at pre assembled hub height is a
prerequisite for the evaluation of wind farm power generation. In the feasibility study
of wind farm, wind turbine power generation should be calculated according to the
wind speed and wind directions at the height of the wind turbine hub. But in the actual
wind measurement, the height of the wind measuring instrument can not completely
meet the installation height of the wind turbine. Therefore, when estimating the gen-
erating capacity of a wind turbine, it is necessary to use wind shear index to extrapolate
the wind speed at any height of the near ground according to the actual wind mea-
surement data of the wind tower [1, 7]. The measured wind speed is as close as possible
to the wind speed at the hub height. For the existing practice, the wind shear index is
calculated according to the measured wind speed at 2 different heights. However, this
method may result in large errors in the calculation results. The main reason is that the
wind shear index is only considered in the neutral state of the atmosphere, without
considering the impact of atmospheric stability on the wind shear index.
© Springer Nature Singapore Pte Ltd. 2017
K. Li et al. (Eds.): LSMS/ICSEE 2017, Part III, CCIS 763, pp. 3–11, 2017.
DOI: 10.1007/978-981-10-6364-0_1
4 H. Lv and G. Chen
The wind shear index is affected by the atmospheric stability, which has been
proved by more and more domestic and foreign scholars. It is pointed out in the
document [2] that when the atmosphere is stable, the speed of wind speed increasing
with the increase of height is faster than logarithmic relationship in the neutral atmo-
sphere. Based on the M-O similarity theory and a series of studies, Panofsky and
Dutton proposed an empirical formula for evaluating the wind shear index using the
atmospheric stability function and the roughness. The wind shear index calculated by
the empirical formula is more in line with the actual situation. The atmosphere is from
stable to neutral to unstable, and the wind shear index decreases in turn [3]. However, it
is a very complex task to obtain the wind shear index when considering the atmo-
spheric stability. Therefore, this method has not been applied in engineering practice.
Aiming at these problems, this paper puts forward the use of Extreme Learning
Machine (ELM) to fit the relationship between wind speed at each height. In this paper,
the wind speed extrapolated by ELM and traditional methods is compared with the
measured wind speed and the vertical extrapolation of wind speed is studied.
2 Wind Shear Index
The wind shear index is a comprehensive parameter to characterize the variation of
wind speed with height, atmospheric stability, surface roughness and so on. In practical
engineering applications, the wind shear index is obtained by only considering the
atmosphere in a neutral state. At this time, the turbulence will depend entirely on the
dynamic factors, and the variation of wind speed with height follows Prandtl’s
empirical formula:
u z
uðzÞ ¼ ln ð1Þ
k z0
In the formula, u is the friction velocity; k is the von Karman constant, k ¼ 0:4.
Friction velocity is defined as: u ¼ ðs=qÞ1=2 , s is the surface shear stress, q is the air
density.
The power exponent formula is derived as follows:
a
z2
u2 ¼ u 1 ð2Þ
z1
a ¼ lnðu1 =u2 Þ= lnðz2 =z1 Þ ð3Þ
In the formula, u2 and u1 are wind speeds at height z2 and z1 , a is the wind shear
index.
In the flat interior, the increase of wind speed with height is submitted to exponential
law. But in some areas affected by terrain factors, the wind speed will reach the peak
value at a certain height, and the wind shear index may even be negative. The main
reason for this phenomenon is the terrain effect. When the airflow through the moun-
tains, due to the obstruction of the terrain, the airflow changes, some of the airflow flows
over the top of the mountain, some of the air flows around both sides of the mountain.
Research on Wind Speed Vertical Extrapolation Based on ELM 5
The more unstable the atmosphere, the more the air flows over, the less the air flows
around. There is a updraft in the windward side of the mountain. At the top of the hill
and on both sides, as the streamline is dense, the wind speeds up. On the leeward side of
the mountain, the wind speed is weakening due to streamline divergence [4].
Based on the M-O similarity theory and a series of studies, Panofsky and Dutton
proposed an empirical formula for evaluating the wind shear index using the atmo-
spheric stability function and the roughness [6, 8]:
a ¼ /m ðz=LÞ=½lnðz=z0 Þ wm ðz=LÞ ð4Þ
In the formula, z is height, L is M-O length, /m and wm are M-O functions.
(Stable atmosphere: /m ¼ 1 þ 5 ðz=LÞ; Unstable atmosphere: /m ¼ ½1 16
ðz=LÞ1=4 ; Neutral atmosphere: /m ¼ 1).
3 Extreme Learning Machine
3.1 ELM Principle
ELM is developed from a single hidden layer feed forward neural network (SLFN) [5].
ELM has many advantages: fast learning speed; less intervention. The weights between
the input layer and the hidden layer of the ELM and the bias of the hidden layer neuron
are given randomly, and do not need to adjust during the training process, which
greatly improves the training time of the algorithm. Set N different random samples
(x i ; t i ), x i ¼ ½xi1 ; xi2 ; . . .xin T 2 Rn , t i ¼ ½ti1 ; ti2 ; . . .tim T 2 Rn . Single hidden layer
* * * *
node number is N ~ and the excitation function is gðxÞ. Standard SLFN model:
~ ~
X
N X N
bi gi xj ¼ bi g ai xj þ bi j ¼ 1; . . .; N ð5Þ
i¼1 i¼1
In the formula, ai ¼ ½ai1 ; ai2 ; . . .ain T is the input weight vector that connects the
*
*
first i hidden layer node; bi is the threshold of the first i hidden layer node; bi ¼
½bi1 ; bi2 ; . . .bim T is the output weight vector that connects the first i hidden layer node;
* *
ai xj represents the inner product of ai and x j .
Standard SLFN can zero error approximate N training samples, and the presence of
~ * *
bi , ai , x j can make the following formula true:
~
X
N
bi g ai xj þ bi ¼ tj ; j ¼ 1; . . .; N ð6Þ
i¼1
Formula (6) written in matrix form:
Hb ¼ T ð7Þ
6 H. Lv and G. Chen
In the formula: H is the hidden layer output matrix. Its row i represents the hidden
layer output of the first i training sample, column j represents the hidden layer output of
the first j hidden layer node of the relative input x1 ; x2 ; ; xN .
2 3 2 3
Gða1 ; b1 ; x1 Þ ... GðaN~ ; bN~ ; x1 Þ gða1 x1 þ b1 Þ ... gðaN~ x1 þ bN~ Þ
6 Gða1 ; b1 ; x2 Þ ... GðaN~ ; bN~ ; x2 Þ 7 6 gða1 x2 þ b1 Þ ... gðaN~ x2 þ bN~ Þ 7
6 7 6 7
H¼6 .. .. .. 7 ¼ 6 .. .. .. 7
4 . . . 5 4 . . . 5
Gða1 ; b1 ; xN Þ . . . GðaN~ ; bN~ ; xN Þ ~
NN gða1 xN þ b1 Þ ... gðaN~ xN þ bN~ Þ ~
NN
2 3 2 3
b11 b12 b1m t11 t12 t1m
6 b21 b22 b2m 7 6 t21 t22 t2m 7
6 7 6 7
b¼6 . .. .. .. 7 ; T ¼ 6 .. .. .. .. 7
4 .. . . . 5 4 . . . . 5
bN1
~ bN2
~ bNm
~ ~
Nm
tN1 tN2 tNm Nm
H and T are determined by the matrix, so the learning process of ELM is the
process of solving the output weights b. According to the document [5], the formula (7)
can be expressed as:
^b ¼ H þ T ð8Þ
In the formula: H þ is a generalized inverse matrix of the matrix H of the hidden
layer.
3.2 Establishment of ELM Model
The purpose of this paper is to solve the problem of vertical extrapolation of wind
speed. The ELM model creation process can be described as: First, two different height
wind speed data at the same time are used as training sets for ELM training. Then, the
wind speed data at a certain height during a certain period are used as testing sets for
ELM testing, and the results are the fitting wind speed of the required height at the
same time. Finally, the wind speed is compared with the actual wind speed, and the
performance of ELM is evaluated. Specific steps are shown in Fig. 1:
training set / test ELM create / ELM simulation performance
set generation train test evaluation
Fig. 1. Modeling steps
The training and testing procedures for ELM are shown in Figs. 2 and 3
respectively:
Research on Wind Speed Vertical Extrapolation Based on ELM 7
invoke the ELM training func on Invoke the ELM tex ng func on
elmtrain elmpredict
Enter a training set (wind speed data at two Enter the test set (the wind speed data at a
different heights over the same period) given height for a given period of me)
Determine the number of neurons in hidden Invoke the elmtrain func on to return the
layer and set the input weight and the threshold input weights, the threshold of the hidden layer
of hidden layer nodes randomly node, and the output weights
Select the hidden layer excita on func on 'sig' Select the hidden layer excita on func on 'sig'
and set the ELM applica on type to 0 and set the ELM applica on type to 0
Calculate hidden layer output matrix H Calculate hidden layer output matrix H
Obtain the predicted wind speed of the required
Calculate output layer weights
height at the same me
End End
Fig. 2. ELM training flow chart Fig. 3. ELM testing flow chart
4 Case Study and Analysis
4.1 Data Sources
The object of this case is a wind farm in Kaifeng Weishi of Henan, which belongs to
the mountainous terrain. According to the wind data of the wind farm provided by a
certain wind power company, some measured wind speed data of No.1 wind tower are
selected as the original data. The chosen time is from March 1, 2013 to February 28,
2014. The height options are 70 m, 50 m and 10 m. The anemometer outputs data
every 10 min. The complete rate of wind data is 99.7% and the integrity is good.
4.2 Annual Wind Shear Index of Wind Farm
By calculation, the annual mean wind speed of each height is shown in Table 1:
Table 1. Annual mean wind speed at each height
Height (m) Annual mean wind speed (m/s)
70 5.012
50 4.397
10 2.609
8 H. Lv and G. Chen
The annual wind shear index is calculated according to the formula (3). The results
are shown in Table 2:
Table 2. Annual wind shear index at each height
Height (m) Wind shear index
70 m 50 m 10 m
70 – 0.389 0.336
50 0.389 – 0.324
10 0.336 0.324 –
4.3 Seasonal Wind Shear Index of Wind Farm
By calculation, the seasonal mean wind speed of each height is shown in Table 3:
Table 3. Seasonal mean wind speed at each height
Height (m) Seasonal mean wind speed (m/s)
Spring Summer Autumn Winter
70 5.638 4.692 4.835 4.899
50 4.948 4.067 4.236 4.354
10 3.069 2.254 2.360 2.769
The seasonal wind shear index is calculated according to the formula (3). The
results are shown in Table 4:
Table 4. Seasonal wind shear index at each height
Height (m) Wind shear index Wind shear index Wind shear index Wind shear index
(spring) (summer) (autumn) (winter)
70 50 10 70 50 10 70 50 10 70 50 10
70 – 0.388 0.313 – 0.425 0.377 – 0.393 0.369 – 0.351 0.293
50 0.388 – 0.297 0.425 – 0.367 0.393 – 0.363 0.351 – 0.281
10 0.313 0.297 – 0.377 0.367 – 0.369 0.363 – 0.293 0.281 –
4.4 Wind Speed Vertical Extrapolation
Using the following three methods for wind speed vertical extrapolation, and the results
are compared with the measured values. Height is from 50 m to 70 m. The time is:
March 5th 14:50–23:50 (time period one), June 5th 14:50–23:50 (time period two),
September 14th 14:50–23:50 (time period three), December 5th 14:50–23:50 (time
period four).
Research on Wind Speed Vertical Extrapolation Based on ELM 9
(1) Method one: Using the annual mean wind speed data to extrapolate wind speed of
the required height according to formula (2).
(2) Method two: Using the seasonal mean wind speed data to extrapolate wind speed of
the required height according to formula (2).
(3) Method three: Using ELM algorithm to fit wind speed of each height.
4.4.1 Wind Speed Comparison Chart
Two charts of comparison between the extrapolated wind speed and the measured
value are shown as follows:
It can be seen from Figs. 4 and 5 that the wind speed extrapolated by these three
methods can well reflect the measured value. The results of method one and method
two are almost the same, while the wind speed extrapolated by method three (ELM) is
closer to the measured value.
Fig. 4. Comparison of extrapolated wind speed and measured value in the time period two
Gt 1030 gearforce software new free download 32 bit - phrase removed
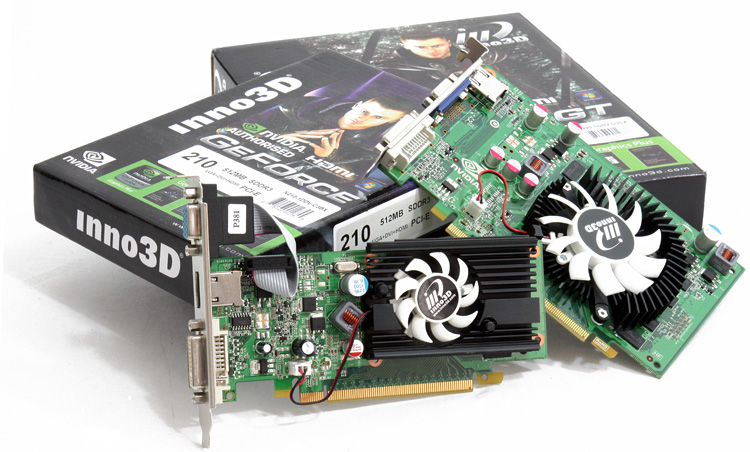
-